
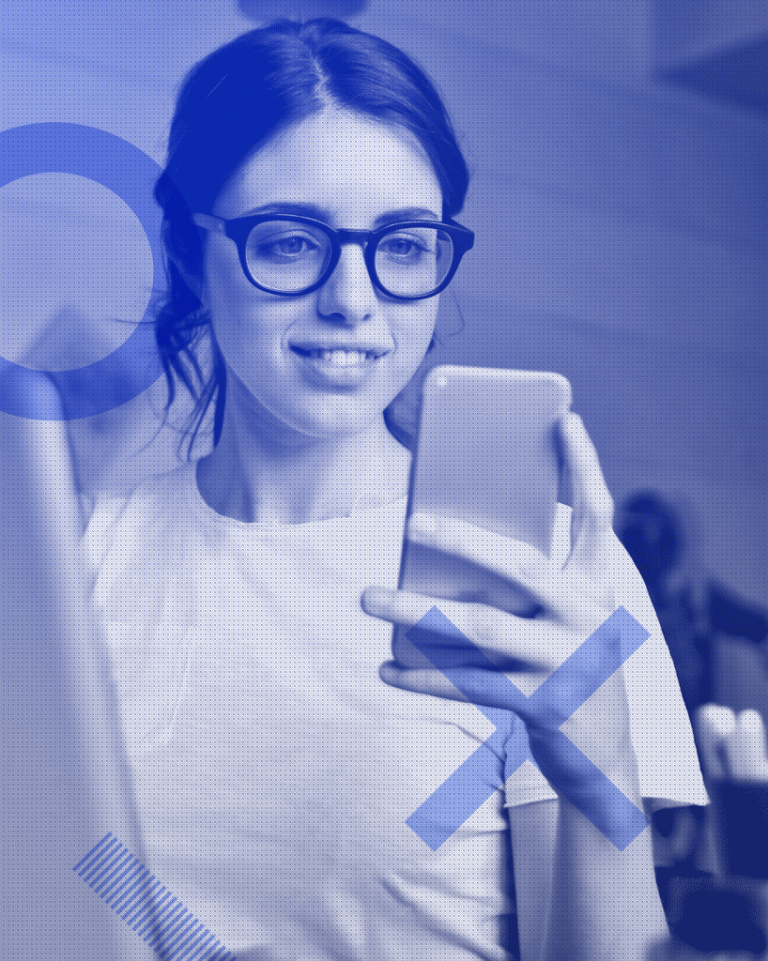
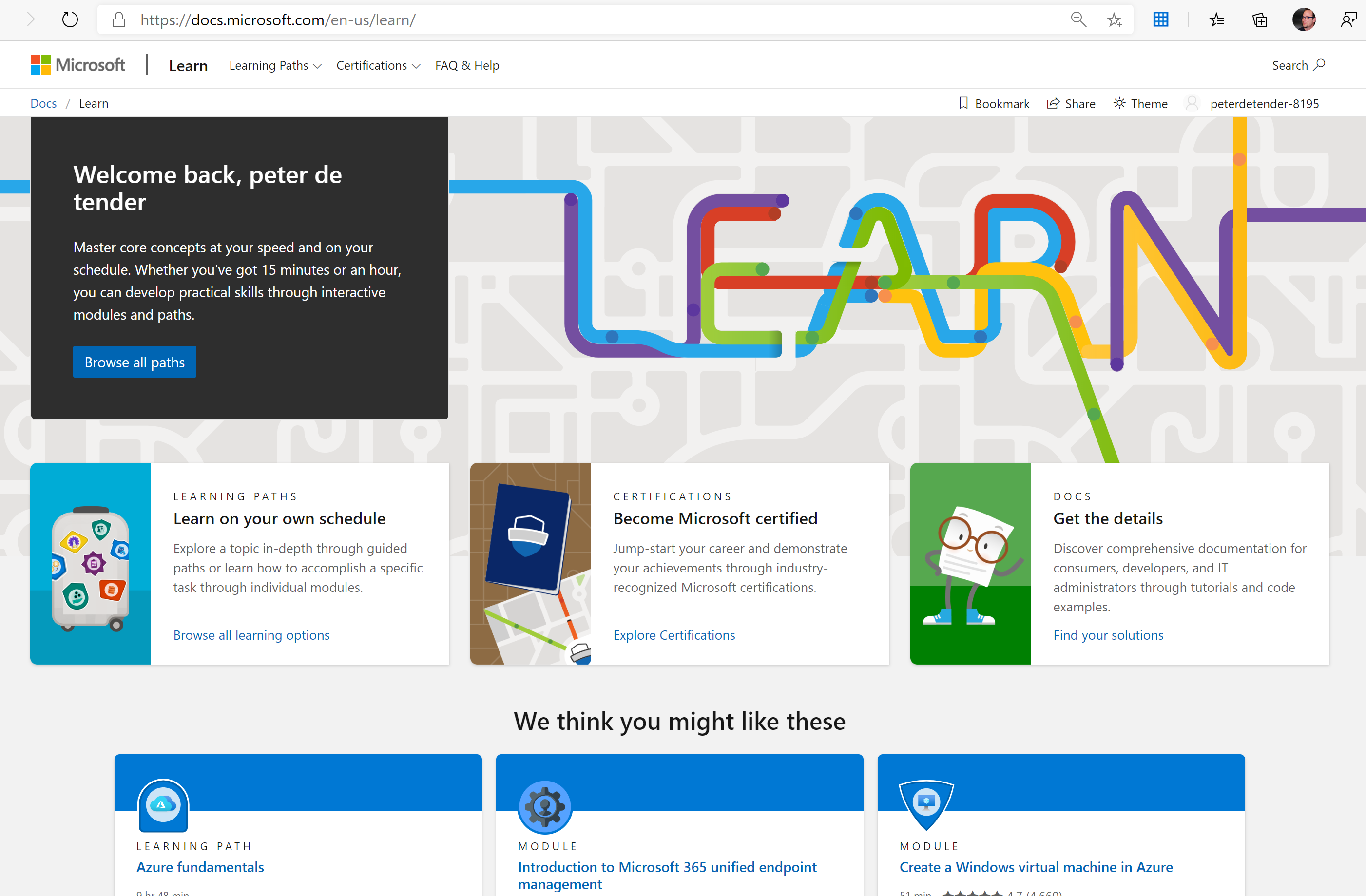
Then, a new neural network with three classifiers is devised to simultaneously determine whether there are fatigue particles, oxide particles and spherical particles in the ferrography image. Firstly, the Inception-v3 model is utilised to extract the features of wear particles automatically.

To this end, this study presents a novel model of wear debris classification to cope with this problem. However, conventional methods of wear debris identification cannot classify wear particles when the wear particles stick together. Combining AdaBN with existing domain adaptation treatments may further improve model performance.įatigue, oxide and spherical particles are the three most common kinds of wear particles in rotate vector (RV) reducer and are often bonded together in ferrography images. Furthermore, we demonstrate that our method is complementary with other existing methods. It archives state-of-the-art performance despite its surprising simplicity. In contrary to other deep learning domain adaptation methods, our method does not require additional components, and is parameter-free. Our approach is based on the well-known Batch Normalization technique which has become a standard component in modern deep learning. In this paper, we propose a simple yet powerful remedy, called Adaptive Batch Normalization(AdaBN), to increase the generalization ability of a DNN. Recent study shows that a DNN has strong dependency towards the training dataset, and the learned features cannot be easily transferred to a different but relevant task without fine-tuning. However, it is still a common (yet inconvenient) practice to prepare at least tens of thousands of labeled image to fine-tune a network on every task before the model is ready to use. Deep neural networks (DNN) have shown unprecedented success in various computer vision applications such as image classification and object detection.
